Data mining is an important role for IT professionals, and a data analytics degree can help you qualify for data mining jobs. But everyone in the business needs to understand data mining, it’s critical to how many business processes are done and how information is collected, so current and aspiring business professionals also need to understand how the process works. Just as finding the right product market is important for businesses, data mining is important for future-ready, self-sustaining businesses. It aids future road planning, product development, and countless business processes that keep the wheels of profit turning. Therefore, in this article, we will address topics related to data mining and business intelligence, the importance of data mining, and how to do data mining to ensure seamless revenue streams.
Table of Contents
What Is Data Mining?
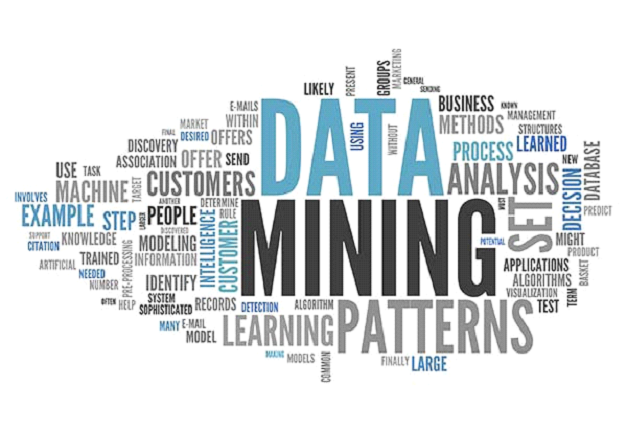
Simply put, data mining is the process by which companies turn raw data into useful information. They use software to find patterns in large volumes of data in order to learn more about customers. It extracts information from datasets and compares them to help businesses make decisions. This ultimately helps them strategize, increase sales, market effectively, and more.
Data mining is sometimes confused with machine learning and data analysis, but these terms are all very different and unique.
While both data mining and machine learning use patterns and analysis, data mining looks for patterns that already exist in data, while machine learning goes beyond predicting future outcomes based on data. In data mining, the “rules” or patterns are not known from the beginning. In many machine learning situations, the machine is given a rule or variable to understand the data. Also, data mining relies on human intervention and decision-making, but machine learning means being initiated by a human and then learning on its own. There is a lot of overlap between data mining and machine learning, and machine learning processes are often used in data mining to automate these processes.
Also, data analysis and data mining are not interchangeable terms. Data mining is used for data analysis, but they are different. Data mining is the process of obtaining information from large data sets, and data analysis is the process by which companies obtain this information and drill down to learn more. Data analysis includes examining, cleaning, transforming, and modeling data. The ultimate goal of the analysis is to discover useful information, draw conclusions, and make decisions.
Data mining, data analytics, artificial intelligence, machine learning, and many other terms are combined in the business intelligence process to help a company or organization make decisions and learn more about its customers and potential outcomes.
What is Data Mining in Business?
The importance of data mining in business is that it is used to transform raw data into meaningful, consumable, actionable insights. Data engineers use software to find patterns that help analyze consumers. Compare datasets with mining relevant metrics that impact revenue lines to follow up on strategies, sales improvement actions, and optimize marketing campaigns.
Due to the overlapping nature of topics between data operations, data mining is often confused and used interchangeably with data analysis and business intelligence. But every term is different.
Data mining refers to the process of extracting information from large datasets, while data analysis refers to the process of discovering patterns from the extracted information. Data analysis includes stages such as examining, cleaning, transforming, and modeling data. The goal is to find information, draw inferences, and take action.
How is Data Mining Used in Business Intelligence?
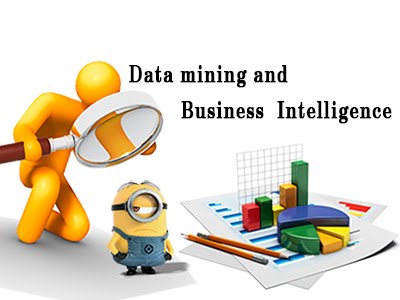
The way we use data mining for business analytics and intelligence varies from one business to another. But there is a structure to this business process management that remains pretty much iron clad. Here’s a look at it.
business understanding
If you are doing data mining for business analysis and want it to be successful, start by identifying the purpose of data mining. Subsequent steps in the plan could address how to use the newly discovered bits of data. Envisioning a data mining algorithm would be a far-fetched task, otherwise, you will briefly stress the purpose of data mining.
Data Understanding
After understanding the purpose of data mining, it is time to touch and feel your data. There are probably as many ways to store and monetize data as there are businesses. How data is created, managed, classified, and commercialized depends on your corporate IT strategy and practices.
Data Preparation
Corporate data is considered to be one of the most important stages in the data mining process for cultivating business intelligence and requires expert handling. Data engineers transform data into a readable format that non-IT professionals can interpret in addition to cleaning and modeling it based on specific attributes.
Data Modeling
Use statistical algorithms to decipher hidden patterns in data. There is a lot of trial and error in finding relevant trends that can improve revenue metrics.
Data Evaluation
The steps involved in data modeling should be assessed for inconsistencies at a micro level. Remember, all roads (must) simplify operations and increase profits.
Implement
The final step is to act on the findings in an observable way. The proposed field trial should be piloted on a smaller scale and then expanded to branch offices after validation.
Data Mining Techniques In Business Analytics.
Now that you understand the importance of data mining, it is very beneficial to understand how data mining works in a business context.
Classification is a more complex data mining technique that uses attributes of data to move them into identifiable categories that help you draw further conclusions. Supermarket data mining can use classification to group the types of food that customers are buying, such as produce, meat, baked goods, etc. These categories can help stores learn more about customers, outputs, and more.
Clustering. This technique is very similar to classification, where data is chunked based on similarity. Clustering groups are less structured than categorical groups, which makes them a simpler option for data mining. In the supermarket example, a simple cluster group could be food and non-food items, rather than a specific class.
Association rules. Associations in data mining are all about tracking patterns, especially those based on linked variables. In the supermarket example, this might mean that many customers who buy a particular item may also buy a second related item. That’s how a store knows how to put certain foods together, or when shopping online, they might display a “people also bought this” section.
Regression analysis. Regression is used in planning and modeling to identify the likelihood of a particular variable. Supermarkets can predict price points based on availability, consumer demand, and competition. Regression aids data mining by identifying relationships between variables in a set.
Anomaly/anomaly detection. For many data mining cases, just seeing the overall pattern may not be all you need. The data also needs to be able to identify and understand outliers data. For example, in a supermarket, if the majority of shoppers were women, but the week in February was mostly men, you would want to investigate this outlier and understand the reasons behind it.
These data mining techniques are key to businesses being able to understand the information they have and improve their practices.
Conclusion
So why is data mining important to businesses? Businesses that utilize data mining can gain a competitive advantage, better understand their customers, have better oversight of business operations, and improve customer acquisition and new business opportunities. Different industries will benefit differently from their data analytics. Some industries are looking for the best way to acquire new customers, others are looking for new marketing techniques, and still, others are working to improve their systems. The data mining process provides businesses with the opportunity and understanding of how to make decisions, analyze information, and move forward.