If you’re managing a wind farm or any other power plant, then you know it well: Maintenance, human error, downtime, and planning inefficiencies can cost millions of dollars each year.
Machine learning and artificial intelligence are the hottest business terms you hear lately.
In fact, renewable energy companies (wind, solar, hydro, nuclear) have benefited greatly from the power of machine learning over the years. They managed to reduce costs, make better forecasts, and improve portfolio returns. And, this trend will only continue at a faster rate. If your company operates in the energy sector or consumes a lot of electricity, machine learning and artificial intelligence are likely to help improve your business performance. But how exactly? Let’s dig a little deeper.
Table of Contents
8 Ways Machine Learning Is Changing Energy
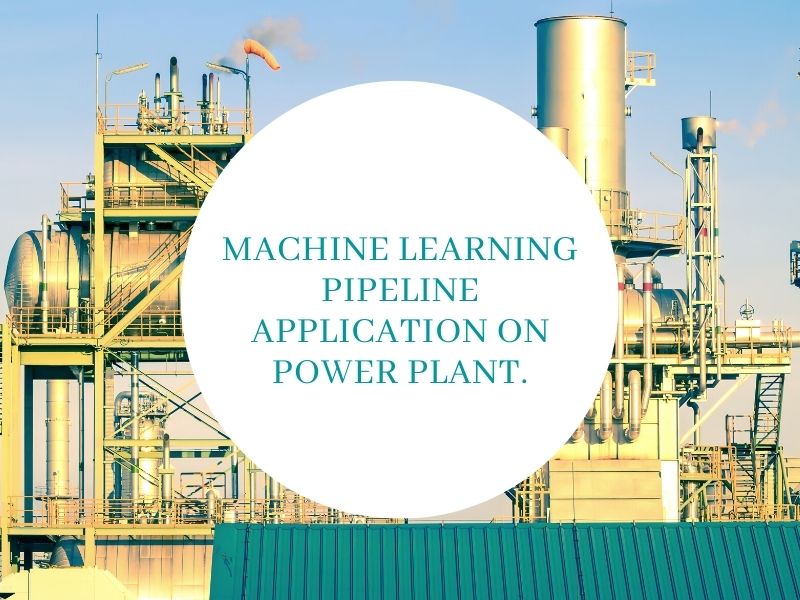
There are many ways machine learning and artificial intelligence can be used to positively transform the energy sector. Below are some of the most popular applications currently in development.
1. Reduce the Potential for Human Error
Human error accounts for 25% of power plant failures each year. Combined with the loss of up to 30 million megawatt-hours of power generation annually, this can lead to customer service disruptions, or worse – think Chornobyl and Three Mile Island. It also means unnecessary costs associated with fixing bugs and bringing the system back online.
To solve this problem, we can use machine learning to support the decision-making of control room operators.
Machine learning provides continuous system monitoring to help you detect anomalies. We also automatically come up with action plans to prevent the situation from getting worse. It can even solve problems before human intervention is required.
This reduces the risk of human error due to distraction, lack of knowledge, or speed of reaction – sometimes the control room operator simply cannot act fast enough to stop a problem.
2. Predict Turbine Malfunction
The wind is a great renewable energy source, but wind turbines are notoriously expensive to maintain. It accounts for 25% of the cost per kWh. And repairing the problem after it occurs can be more expensive.
Machine learning can help you address this problem ahead of time, reducing maintenance costs by spotting problems with turbines before they fail. This is especially important when wind farms are located in inaccessible places, such as in the middle of the ocean, which makes maintenance more expensive.
Real-time data collected through Supervisory Control and Data Acquisition (SCADA) can help to identify possible failures in the system in advance to prevent failures.
For example, we can use data from sensors within turbines, such as oil, grease, and vibration sensors, to train machine learning models to identify precursors to failure, such as low lubricant levels. This method can predict failures up to 60 days in advance.
3. Increase Power Plant Profitability with Optimized Scheduling and Pricing
Volatility in energy prices means that operating a power plant can be more or less profitable, depending on something as simple as the time of day.
But because the utility market is so fast-paced, it can be difficult to manually track all the data needed to make these decisions.
We can use machine learning to help. By feeding historical data on prices and usage into machine learning algorithms, you can predict the best times for your plants to run and make more money. Machine learning can spot when usage is high but the price of raw materials used to produce energy is low. These extremely accurate forecasts create an optimized power generation schedule that maximizes profitability.
If you are working on any of these use cases and need some advice, please contact us – we have helped E.ON, Danske Commodities, Rheingau and other utilities optimize profitability with custom machine learning software.
4. Energy Consumption
Switching to renewable energy is not just a concern for governments and power companies. In fact, many companies like Google and Microsoft are trying to impact the environment and their bottom lines by reducing their overall energy consumption.
Google is known for the huge data centers it builds around the world. These data centers generate a lot of heat and require a lot of electricity to cool.
To solve this problem, DeepMind AI used machine learning algorithms to reduce the energy consumption of its Google data centers by 40%. In addition to drastically reducing their utility bills, this also helps lower overall emissions, reducing the carbon tax they would otherwise have to pay.
5. Predictive Maintenance
In addition to helping match energy production with energy consumption, AI is becoming a major driver in ensuring grid reliability and robustness.
In 2003, a major power outage in Ohio was caused by a low-hanging high-voltage power line brushing against an overgrown tree. The power system alarm failed and there is no indication that the incident has occurred. The utility didn’t notice anything until three other wires started failing for similar reasons. Ultimately, this oversight created a cascading effect that caused the entire grid to collapse.
The power outage lasted for two days and affected 50 million people. In addition, 11 people died and about $6 billion was lost.
6. Energy Source Exploration
n addition to weather forecasting and renewable energy optimization, AI is being used in fossil fuel energy exploration and drilling.
Two years ago, ExxonMobil teamed up with the Massachusetts Institute of Technology (MIT) to produce a self-learning submersible robot for exploring the ocean surface. The robots will be equipped with machine learning algorithms that will not only help them learn from mistakes as they explore, but will do the same work that scientists would do without risk.
Robots will explore, record, and analyze seafloor data to help identify new locations to drill for oil and gas.
7. Grid Management
One of the most interesting applications of AI in the energy sector is grid management. Electricity is delivered to customers through a complex network, also known as the grid. The tricky part about the grid is that the amount of electricity generated and the demand for electricity must always match. Otherwise, problems such as power outages and system failures may occur.
While there are many methods of storing energy, the most common is the old but still effective method of pumped hydro. It works by pumping water to a certain height and then letting it fall on a turbine, where it can be used again.
When dealing with renewable energy, it is difficult to predict the power generation capacity of the grid. After all, it depends on several factors, such as sunlight and wind.
8. Demand Response
When demand fluctuates wildly, the costs can be prohibitively high for countries that generate most of their energy from renewable sources. As most countries turn to green energy, it becomes more difficult to effectively deal with fluctuations in demand. For example, Germany plans to cover 80% of its electricity consumption with renewable energy by 2050.
A country like Germany will face two main problems. First, demand fluctuates. It is not uncommon for electricity demand to spike on a certain day of the year or during a certain period of time, such as Christmas. The second problem is weather fluctuations. If there is no wind, or if the sky is cloudy, it may be difficult to meet power needs.
The Future of Machine Learning in Energy
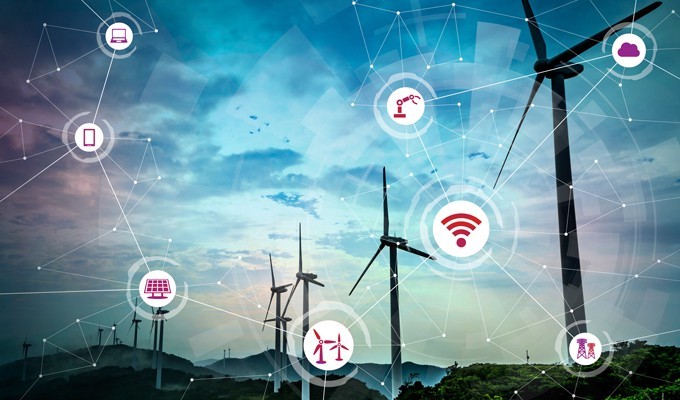
Machine learning and artificial intelligence certainly have a long way to go in the energy sector. As developed countries pursue a fully green economy, maintaining a balanced, resilient, and reliable grid is a priority.
This is where the smart grid comes into play. A smart grid is an electrical grid that combines the power of IoT and artificial intelligence to create a digital grid that enables two-way communication between consumers and utilities.
Smart grids are equipped with smart meters, sensors, and alarm devices that continuously collect and display data to consumers, thereby improving consumers’ energy consumption behavior. It can also be fed back to machine learning algorithms to predict demand, improve performance, reduce costs and prevent system failures.
While some developed countries are adopting smart grids, we still have a long way to go before moving to 100% renewable energy, AI-controlled distribution, and grid management.
Final Words
The advent of machine learning and artificial intelligence has greatly expanded the possibilities; however, there is a lot to be said for partnering with the right experts to ensure the most accurate, insightful, and actionable information from data.
This is why choosing the right data science platform is so important. RapidMiner unifies the entire data science lifecycle from data preparation to machine learning to predictive model manipulation.
We can help you maximize efficiency to meet energy demands and better serve your customers.