Machine learning can be used in Customer Insights to find sophisticated patterns across large data sets. It’s critical to comprehend the process by which these patterns appear: algorithms look at past data to find trends that can be applied to fresh data in the future.
Around 2.5 quintillion bytes of data are produced daily by customers all over the world in the modern era. Nearly all of the time throughout the day, a customer produces data.
Table of Contents
Application Of Machine Learning In Customer Analytics
Machine learning is one of the key tools used in customer analytics and in this article we will see 5 applications:
- Customer segmentation
- Sentiment Analysis
- Customer churn prediction
- Recommendation system
- Customer service
1. Customer Segmentation
The traits and behaviors of every customer are distinctive. But among the vast amount of data, there are also some similarities that can be found. We can segment the customers using a variety of factors, including demographics, geography, behavior, need, and value. These segments assist in identifying specific customer behavior patterns, which in turn enables us to better understand the needs of various customer segments, develop targeted marketing strategies, and increase customer engagement.
But with millions of rows of data, how can we segment the customers? We employ techniques for machine learning! Similar groups within the data can be found using clustering algorithms like K-Means. So that we can send relevant advertisements, promotions, or suggestions, we can understand the various groups.
2. Sentiment Analysis
Your product has officially launched after months of planning, designing, and production. How, though, can you tell what your customers are saying about your offering? Customer sentiment analysis is one method of comprehending this. We end up with a lot of text data after gathering customer reviews from online stores, websites, phone calls to customer service, and customer satisfaction surveys. Natural language processing can be used to determine the aspects of the product that customers are discussing as well as whether their opinions are positive, neutral, or negative.
The voice of the customer is very important as understanding your customer this way helps tweak the product or service, understand what makes your customer happy or enhance brand perception among the competitors.
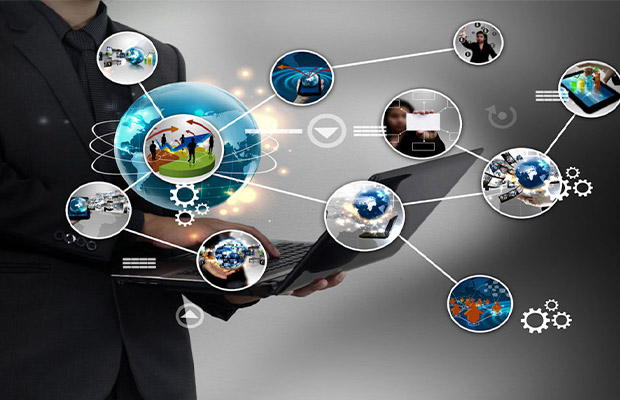
3. Customer Churn Prediction
Five times as much money goes into acquiring a new customer as into keeping an existing one. Therefore, the primary objective of customer churn prediction is to aid in customer retention, which in turn aids in boosting the company’s revenue. A customer’s churn data is gathered through either their prolonged inactivity or their cancellation of a product or service.
Therefore, we can predict whether a customer will likely churn or not using binary classification methods like logistic regression. If a customer appears to be at risk of leaving, we can further investigate them to determine which market segment they fall under and take the appropriate action to keep them.
4. Recommendation System
When we visit a store and are helped by a store clerk, we not only find what we’re looking for but the clerk also makes an effort to cross-sell or upsell their goods. This is comparable to what a recommendation system does when a customer makes an online purchase. Personalization, revenue, and customer satisfaction are all goals of recommendation engines. This not only aids in the customer’s discovery of new goods but also increases sales for the business.
The development of collaborative filtering, content-based filtering, and hybrid recommendation systems using customer purchasing and behavior data and identification of similarities with other customer purchases are some ways to implement such a system.
5. Customer Service
The time of a customer is extremely valuable. There will be several times when a customer needs support while working with a company, a product, or a service. We may compile information on the nature of support calls, chatbot interactions, frequency of customer visits to the support page, or in-person visits for offline support. This will make it easier to determine where a customer needs the most assistance.
Regression analysis can be used to find trends in these variables and forecast potential problems or suggest ways to decrease customer complaints. This would improve customer experience and lower customer churn.
Conclusion
Remember, artificial intelligence is not a cure-all. Machine learning in customer analytics can help you understand your customers better, but it’s far from perfect. Accuracy issues can arise from noisy data or misinterpreted algorithms.
However, machine learning holds real promise as the way to approach analytics in the future. When no other method of solution is available, it can solve challenging issues. For those who adopt it, it offers the knowledge and potential to quickly update corporate beliefs and take advantage of real-time information, giving them a competitive advantage.
Even though machine learning is still far from perfect, it has its uses and is unquestionably worth implementing for bigger gains.
More To Explore: